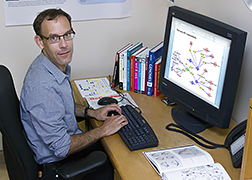
Software for Teasing Out Food Compound Benefits
By Jan Suszkiw
July 15, 2020
A team of Agricultural Research Service (ARS) scientists and their collaborators has leveraged the power of machine-learning technology to speed the identification of health-promoting compounds in food.
The chemical make-up and dietary importance of essential nutrients like vitamin A and riboflavin are well-defined. However, there are thousands of other food compounds whose biological activity and effects in the human body over a lifetime of exposure through diet are poorly understood.
This knowledge gap is referred to as the dark matter of the human "exposome," explained Laurence Parnell, a computational biologist with the USDA Jean Mayer Human Nutrition Research Center on Aging (JMHNRCA), operated jointly by ARS and Tufts University in Boston, Massachusetts.
To shed light on this so-called dark matter, Parnell and colleagues developed a software program called PhyteByte. It uses machine-learning algorithms and sophisticated decision trees to predict the biological activity of these mystery food compounds from masses of database information.
PhyteByte taps into two databases. The first, called FooDB, currently contains a catalog of 70,926 compounds in food, including plant pigments thought to confer health benefits, like cyanidin 3-(6''-acetyl-galactoside) from blueberries and quercetin 3,4',7-triglucoside in garden onions and red wine.
The second database is ChEMBL. It stores information on the chemical properties of nearly 2 million molecules, including pharmaceutical drugs like thiazolidinedione, which is widely used to treat type-2 diabetes. The database also is an important resource because it contains information on biological activity for many compounds.
A PhyteByte session begins with inputting the name of a protein of interest, typically one that is the target of prescribed medicines. The software then queries ChEMBL for chemical structures that are similar to the drug target of the protein of interest. Then, the machine learning part of the algorithm builds a sophisticated model that's used to "pull" from FooDB a set of highly similar food compounds, along with a list of foods containing the compound and in what amount.
PhyteByte's predictions aren't intended as stand-alone evidence, however. Rather, they're meant to help researchers prioritize which food compounds are the best candidates for conducting actual laboratory research and clinical studies, which can be time-consuming and costly.
Ultimately, the knowledge gleaned from research on these candidate compounds will contribute to improved dietary recommendations and planning—such as by nutritionists—as well as new insight into the genetic responses of individual consumers to certain foods and food-drug interactions, according to Parnell.
He recently co-authored a paper on PhyteByte's potential in the journal BMC Bioinformatics together with colleagues Kenneth Westerman of Massachusetts General Hospital, Sean Harrington of Notemeal, Inc.; and Josè Ordovás of the JMHNRCA's Nutrition and Genomics Laboratory.
The Agricultural Research Service is the U.S. Department of Agriculture's chief scientific in-house research agency. Daily, ARS focuses on solutions to agricultural problems affecting America. Each dollar invested in agricultural research results in $20 of economic impact.